Technology in Quality Control: A Modern Approach
Technology in quality control has revolutionized how businesses ensure the quality of their products and services. Gone are the days of manual inspections and subjective assessments. Today, advanced technologies like […]
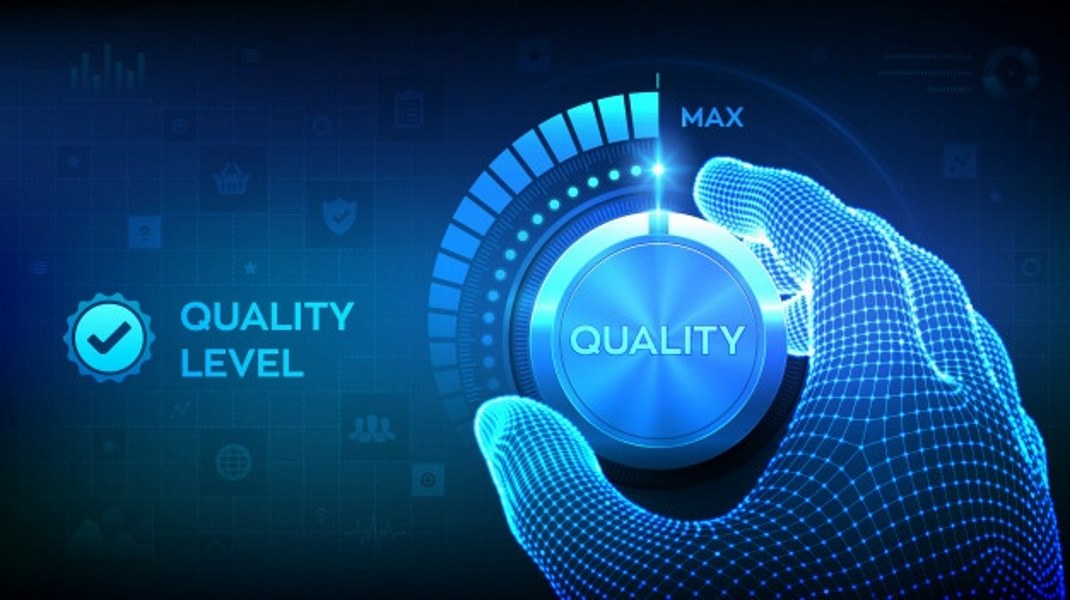
Technology in quality control has revolutionized how businesses ensure the quality of their products and services. Gone are the days of manual inspections and subjective assessments. Today, advanced technologies like automation, data analytics, and artificial intelligence are transforming quality control processes, leading to increased efficiency, accuracy, and consistency.
This evolution has brought significant benefits, including reduced costs, improved customer satisfaction, and faster time-to-market. By leveraging these technological advancements, organizations can proactively identify and address quality issues, ultimately delivering superior products and services.
Introduction to Technology in Quality Control
Quality control (QC) has always been a critical aspect of manufacturing and service industries, ensuring that products and services meet predetermined standards. Over time, QC practices have evolved, adapting to technological advancements and changing customer demands.
Technology has revolutionized the way quality is managed in modern businesses, enabling more efficient, data-driven, and comprehensive approaches to QC.
The Evolution of Quality Control Practices
The evolution of QC practices can be traced back to the early days of industrialization, where quality was primarily assessed through manual inspection. This traditional approach was often subjective and prone to human error.
With the advent of the Industrial Revolution, the need for standardized and systematic QC practices became increasingly apparent. This led to the development of statistical process control (SPC) methods, which introduced statistical tools and techniques to monitor and control manufacturing processes.
The rise of computers in the latter half of the 20th century further transformed QC practices. Computers enabled the automation of data collection, analysis, and reporting, making QC more efficient and data-driven.
The Role of Technology in Modern Quality Control Systems
Modern QC systems leverage a wide range of technologies, including:
- Data Acquisition Systems: Sensors, cameras, and other data acquisition devices collect real-time data on various process parameters, such as temperature, pressure, and dimensions.
- Data Analytics: Statistical software and machine learning algorithms analyze vast amounts of data to identify trends, patterns, and anomalies that may indicate quality issues.
- Automated Inspection Systems: Vision systems, robots, and other automated inspection technologies can perform repetitive and complex inspections with high accuracy and speed.
- Quality Management Software: Software platforms streamline QC processes, manage documentation, track defects, and facilitate communication and collaboration among stakeholders.
- Internet of Things (IoT): Connected devices and sensors enable real-time monitoring of production processes and equipment, allowing for proactive identification and resolution of potential quality issues.
Benefits of Integrating Technology in Quality Control
Integrating technology into QC systems offers numerous benefits, including:
- Improved Accuracy and Precision: Automated inspection and data analysis eliminate human error and subjectivity, leading to more accurate and precise quality assessments.
- Enhanced Efficiency: Automation and data-driven decision-making streamline QC processes, reducing inspection time and improving overall efficiency.
- Proactive Quality Management: Real-time data analysis allows for early detection of potential quality issues, enabling proactive corrective actions and preventing defects.
- Data-Driven Decision-Making: Access to comprehensive data provides insights into process performance, allowing for informed decision-making to optimize quality and reduce costs.
- Increased Customer Satisfaction: Consistent product quality and reduced defects lead to higher customer satisfaction and improved brand reputation.
Key Technologies in Quality Control
Quality control has evolved significantly with the advent of technology. These advancements have not only improved the efficiency and effectiveness of quality control processes but also opened up new possibilities for data analysis, automation, and real-time monitoring.
Statistical Process Control (SPC)
Statistical process control (SPC) is a powerful tool for monitoring and controlling processes based on statistical analysis. SPC helps identify trends, variations, and potential issues within a process, allowing for timely interventions to maintain quality.
- Control Charts: Control charts are visual representations of data points collected over time, plotted against predetermined control limits. These charts help identify trends, shifts, and outliers, indicating potential process variations.
- Process Capability Analysis: This analysis assesses the ability of a process to meet specified quality requirements. It determines the process’s potential for producing consistent output within the desired tolerances.
- Acceptance Sampling: This method involves inspecting a random sample of products from a batch to assess the quality of the entire batch. It’s used when inspecting the entire batch is impractical or expensive.
SPC is widely used in manufacturing, healthcare, and service industries to monitor and improve processes, reduce defects, and ensure consistent quality. For example, in manufacturing, SPC is used to track and control variables like temperature, pressure, and dimensions during production, ensuring products meet specifications. In healthcare, SPC is used to monitor infection rates, patient wait times, and other key metrics to improve patient safety and care quality.
Automated Inspection Systems, Technology in quality control
Automated inspection systems utilize advanced technologies like computer vision, machine learning, and robotics to automate the inspection process. These systems can identify defects, inconsistencies, and deviations from predetermined standards, enhancing accuracy and efficiency.
- Computer Vision: Computer vision algorithms analyze images and videos to detect defects, measure dimensions, and identify patterns. They can be used for tasks like surface inspection, dimensional measurement, and object recognition.
- Machine Learning: Machine learning algorithms can be trained on large datasets of defect images to identify and classify defects automatically. They can adapt to new defect types and improve their accuracy over time.
- Robotics: Robots can be used to perform automated inspection tasks, especially in hazardous or repetitive environments. They can access hard-to-reach areas and perform inspections with precision and consistency.
Automated inspection systems are widely used in industries like automotive, electronics, and food processing to improve inspection accuracy, reduce human error, and increase throughput. For example, in automotive manufacturing, automated inspection systems are used to inspect car bodies for paint defects, scratches, and dents. In electronics manufacturing, they are used to inspect circuit boards for solder defects and component placement errors.
Data Analytics and Big Data
Data analytics plays a crucial role in quality control by providing insights into process performance, identifying potential issues, and predicting future outcomes. Big data technologies enable the collection, storage, and analysis of vast amounts of data, allowing for more comprehensive and insightful analysis.
- Predictive Analytics: Predictive analytics uses historical data to predict future outcomes, allowing for proactive measures to prevent defects and ensure quality.
- Root Cause Analysis: Data analytics can be used to identify the root causes of quality issues, enabling targeted improvements and preventing recurrence.
- Process Optimization: Data analysis can help identify areas for process improvement, leading to increased efficiency, reduced waste, and improved quality.
Data analytics is used in various industries, including manufacturing, healthcare, and finance, to improve quality control processes. For example, in manufacturing, data analytics can be used to predict machine failures, optimize production schedules, and identify trends in defect rates. In healthcare, it can be used to analyze patient data to identify risk factors for diseases, improve patient care, and optimize resource allocation.
Quality Management Software (QMS)
Quality management software (QMS) provides a centralized platform for managing quality control processes, documentation, and data. It helps organizations streamline quality control activities, improve communication, and ensure compliance with industry standards.
- Document Management: QMS systems allow for the creation, storage, and management of quality-related documents, including procedures, specifications, and audit reports.
- Non-Conformance Tracking: QMS systems facilitate the tracking and resolution of non-conformance issues, ensuring prompt corrective actions are taken.
- Auditing and Reporting: QMS systems provide tools for conducting audits and generating reports on quality performance, helping organizations identify areas for improvement.
QMS systems are used by organizations in various industries, including manufacturing, healthcare, and pharmaceuticals, to improve quality control processes, enhance compliance, and reduce risk. For example, in manufacturing, QMS systems can be used to manage supplier audits, track product defects, and generate reports on quality performance. In healthcare, they can be used to manage patient records, track medical device recalls, and ensure compliance with regulatory standards.
Internet of Things (IoT)
The Internet of Things (IoT) enables the collection of real-time data from sensors and devices connected to the internet. This data can be used to monitor quality parameters, identify potential issues, and optimize processes in real time.
- Real-time Monitoring: IoT sensors can monitor critical parameters like temperature, pressure, and vibration, providing real-time insights into process performance.
- Predictive Maintenance: By analyzing sensor data, IoT can predict potential equipment failures, enabling preventive maintenance and reducing downtime.
- Process Optimization: IoT data can be used to optimize processes by identifying bottlenecks, inefficiencies, and areas for improvement.
IoT is increasingly used in industries like manufacturing, logistics, and agriculture to improve quality control processes. For example, in manufacturing, IoT sensors can be used to monitor production lines, detect defects, and trigger automated corrective actions. In logistics, IoT can be used to track shipments, monitor temperature and humidity, and ensure product integrity throughout the supply chain.
Blockchain Technology
Blockchain technology provides a secure and transparent platform for tracking products and materials throughout their lifecycle. It can be used to enhance transparency, traceability, and accountability in quality control processes.
- Supply Chain Transparency: Blockchain can track the origin, movement, and history of products and materials, providing a complete and auditable record of their journey.
- Product Authentication: Blockchain can be used to authenticate products, preventing counterfeiting and ensuring authenticity.
- Quality Data Management: Blockchain can store and manage quality data, ensuring its integrity and immutability.
Blockchain technology is being explored for its potential to improve quality control in various industries, including food, pharmaceuticals, and luxury goods. For example, in the food industry, blockchain can be used to track the origin of food products, ensuring their safety and traceability. In pharmaceuticals, it can be used to track the movement of drugs, preventing counterfeiting and ensuring their authenticity.
Artificial Intelligence (AI)
Artificial intelligence (AI) is transforming quality control by automating tasks, analyzing data, and identifying patterns that are difficult for humans to detect. AI algorithms can be used to improve defect detection, predict quality issues, and optimize processes.
- Defect Detection: AI algorithms can be trained on large datasets of defect images to identify and classify defects with high accuracy.
- Predictive Quality Control: AI can analyze historical data to predict potential quality issues, allowing for proactive measures to prevent defects.
- Process Optimization: AI can analyze process data to identify bottlenecks, inefficiencies, and areas for improvement, leading to optimized processes and improved quality.
AI is being implemented in various industries, including manufacturing, healthcare, and finance, to improve quality control processes. For example, in manufacturing, AI can be used to inspect products for defects, predict machine failures, and optimize production schedules. In healthcare, AI can be used to analyze medical images, diagnose diseases, and personalize patient care.
Automation in Quality Control
Automation has revolutionized quality control processes, bringing significant improvements in efficiency, accuracy, and consistency. Automation tools and technologies have replaced manual tasks, resulting in faster turnaround times, reduced errors, and enhanced product quality.
Benefits of Automated Quality Control Systems
Automation offers several benefits for quality control processes. Here are some of the key advantages:
- Increased Efficiency: Automated systems can perform tasks much faster than humans, leading to faster turnaround times and increased productivity. This is particularly beneficial for repetitive and time-consuming tasks such as data entry, inspection, and testing.
- Improved Accuracy: Automated systems are less prone to human error, resulting in more accurate data collection and analysis. This ensures that quality control decisions are based on reliable information, leading to better product quality.
- Enhanced Consistency: Automated systems can perform tasks consistently, regardless of operator fatigue or variability. This ensures that quality control processes are standardized and repeatable, leading to consistent product quality.
- Reduced Costs: Automation can help reduce labor costs by automating repetitive tasks. This can also lead to cost savings through reduced errors and improved efficiency.
- Improved Data Analysis: Automated systems can collect and analyze large amounts of data, providing valuable insights into product quality and performance. This data can be used to identify trends, predict potential problems, and improve quality control processes.
Examples of Automated Quality Control Systems
Automated quality control systems are widely used in various industries. Here are some examples:
- Automated Vision Inspection Systems: These systems use cameras and image processing software to inspect products for defects. They are commonly used in manufacturing industries to inspect products for surface defects, dimensional accuracy, and other quality issues. Automated vision inspection systems can be used to inspect a wide range of products, including electronics, automotive parts, and food products.
- Automated Measurement Systems: These systems use sensors and software to measure product dimensions, weight, and other parameters. They are commonly used in industries such as manufacturing, pharmaceuticals, and aerospace to ensure that products meet specified tolerances. Automated measurement systems can be used to inspect products for dimensional accuracy, weight, and other critical parameters.
- Automated Testing Systems: These systems use automated equipment to perform tests on products, such as pressure tests, temperature tests, and electrical tests. They are commonly used in industries such as automotive, electronics, and aerospace to ensure that products meet safety and performance standards. Automated testing systems can be used to perform a wide range of tests, including functional tests, performance tests, and safety tests.
Data Analytics and Quality Control
Data analytics plays a crucial role in modern quality control by providing insights into product performance, identifying potential issues, and enabling proactive measures to enhance quality. By analyzing vast amounts of data collected throughout the production process, businesses can gain a comprehensive understanding of product quality trends, identify areas for improvement, and make informed decisions to optimize their operations.
Identifying Quality Issues
Data analytics can be used to identify quality issues by analyzing data from various sources, such as production records, customer feedback, and sensor readings. This data can be used to identify patterns and anomalies that indicate potential quality problems. For instance, if a spike in customer complaints about a particular product feature is observed, data analytics can be used to investigate the root cause of the issue. This could involve analyzing production data to identify potential defects in the manufacturing process, or examining customer feedback to understand the specific aspects of the product that are causing dissatisfaction.
Predicting and Preventing Defects
Data analytics can be used to predict and prevent defects by identifying patterns and trends in data that suggest potential quality problems. This predictive capability allows businesses to take proactive measures to prevent defects from occurring in the first place. For example, by analyzing historical data on product failures, businesses can identify the factors that are most likely to contribute to defects. This information can then be used to develop preventative measures, such as adjusting production parameters or implementing stricter quality control procedures.
Hypothetical Scenario
Imagine a company that manufactures smartphones. The company has been experiencing a high rate of customer complaints about battery life. To address this issue, the company decides to use data analytics to identify the root cause of the problem. They collect data from various sources, including production records, customer feedback, and sensor readings from the smartphones themselves. By analyzing this data, they discover that a particular batch of batteries from a specific supplier is consistently performing below expectations. Armed with this information, the company can take immediate action to address the issue, such as contacting the supplier to investigate the problem or replacing the defective batteries in existing products. This proactive approach helps to improve product quality and customer satisfaction.
Quality Control in Manufacturing
Quality control in manufacturing is a critical aspect of any production process, ensuring that products meet established standards and customer expectations. The manufacturing environment presents unique challenges for quality control, requiring specialized technologies and strategies to maintain consistency and prevent defects.
Challenges in Manufacturing Quality Control
The manufacturing process involves complex operations, intricate machinery, and diverse materials, creating various challenges for maintaining quality.
- High-volume production: Manufacturing often involves producing large quantities of products, making it difficult to monitor each item individually and ensure consistent quality.
- Process variability: Manufacturing processes are susceptible to variations, including changes in materials, equipment performance, and environmental conditions, which can impact product quality.
- Complex products: Modern manufacturing produces increasingly complex products with intricate designs and multiple components, requiring sophisticated quality control measures to ensure proper assembly and functionality.
- Rapid technological advancements: The rapid pace of technological advancements introduces new materials, processes, and equipment, necessitating continuous adaptation and innovation in quality control strategies.
- Global supply chains: Manufacturing often involves global supply chains, making it challenging to monitor quality throughout the entire production process and ensure consistency across different locations.
Technologies for Quality Control in Manufacturing
To address these challenges, manufacturers have adopted various technologies to enhance quality control, automate processes, and ensure product consistency.
- Automated inspection systems: These systems use advanced sensors, cameras, and image processing algorithms to automatically inspect products for defects, reducing reliance on manual inspection and improving accuracy.
- Statistical process control (SPC): SPC utilizes statistical methods to monitor and analyze manufacturing processes, identifying potential deviations from established standards and allowing for timely adjustments to prevent defects.
- Machine vision: Machine vision systems use cameras and computer algorithms to analyze images and identify defects, allowing for real-time quality control and automated decision-making.
- 3D scanning and metrology: These technologies provide precise measurements of product dimensions and geometry, enabling manufacturers to verify product conformity and identify potential deviations from design specifications.
- Data analytics and predictive modeling: Data analytics tools analyze historical data from manufacturing processes to identify patterns and predict potential quality issues, enabling proactive measures to prevent defects and improve overall quality.
- Internet of Things (IoT): IoT sensors and devices collect real-time data from manufacturing equipment and processes, enabling continuous monitoring, predictive maintenance, and improved quality control.
- Robotics: Robots can be deployed in manufacturing processes to perform repetitive tasks with high precision and accuracy, reducing human error and improving consistency in product quality.
Implementation Examples
These technologies are widely implemented in real-world manufacturing scenarios to enhance quality control and improve product consistency.
- Automotive industry: Automated inspection systems are used to inspect car bodies for paint defects, ensuring a high-quality finish. 3D scanning is employed to verify the dimensions and alignment of car parts, ensuring proper fit and functionality.
- Electronics manufacturing: Machine vision systems are used to inspect circuit boards for component placement errors, ensuring proper functionality of electronic devices. Data analytics is employed to monitor production processes and identify potential defects, enabling timely interventions to prevent quality issues.
- Pharmaceutical industry: Automated inspection systems are used to inspect tablets and capsules for size, shape, and color uniformity, ensuring product consistency and safety. SPC is employed to monitor production processes and ensure adherence to strict quality standards for pharmaceutical products.
Quality Control in Service Industries
Quality control in service industries is a crucial aspect of ensuring customer satisfaction and building a strong reputation. Unlike manufacturing, where tangible products are inspected, service quality is often subjective and intangible, making its measurement and control more challenging.
Technologies Used for Quality Control in Service Delivery
The unique nature of service industries necessitates the use of specific technologies to effectively monitor and manage quality. These technologies enable service providers to gather real-time data, identify areas for improvement, and ultimately enhance customer experience.
- Customer Relationship Management (CRM) Systems: CRM systems are used to track customer interactions, preferences, and feedback. This data helps service providers understand customer needs, anticipate issues, and personalize service delivery. For example, a CRM system can track customer complaints, identify recurring issues, and trigger automated responses to address common concerns.
- Customer Feedback Platforms: Online surveys, social media monitoring, and feedback apps provide valuable insights into customer satisfaction levels. Service providers can use this feedback to identify areas for improvement and measure the effectiveness of their quality control initiatives. For instance, a restaurant chain might use an online survey to collect feedback on the quality of its food and service, identifying areas where customer satisfaction is low.
- Process Automation Tools: Automation can streamline service delivery processes, reduce errors, and ensure consistency in service quality. For example, a call center might use automated scripts to guide agents through customer interactions, ensuring consistent handling of common queries.
- Real-Time Monitoring and Analytics: Real-time monitoring tools, such as dashboards and performance analytics, allow service providers to track key performance indicators (KPIs) related to service quality. This data can be used to identify trends, spot potential problems, and proactively address issues. For instance, a bank might use real-time monitoring to track wait times at branches, identifying branches with excessive wait times and taking steps to improve service efficiency.
The Future of Technology in Quality Control
The future of quality control is inextricably linked to the advancement of technology. As we move forward, we can expect to see a continued evolution of quality control practices, driven by the integration of cutting-edge technologies and the emergence of new paradigms.
Emerging Technologies and Their Impact
Emerging technologies are poised to revolutionize quality control practices, leading to significant improvements in efficiency, accuracy, and overall product quality.
- Artificial Intelligence (AI) and Machine Learning (ML): AI and ML are transforming quality control by automating tasks, analyzing vast datasets, and identifying patterns that humans might miss. AI-powered systems can analyze real-time data from sensors and cameras, detecting defects and anomalies in products and processes. They can also be used to predict potential issues and proactively adjust production parameters to prevent defects.
- Internet of Things (IoT): The IoT enables the collection and analysis of data from connected devices and sensors throughout the production process. This data can be used to monitor production parameters, identify potential problems, and track product quality in real-time. By connecting devices and systems, the IoT facilitates seamless data sharing and real-time insights, enabling faster and more informed decision-making.
- Robotics and Automation: Robots are increasingly being deployed in quality control tasks, performing repetitive and complex inspections with greater accuracy and speed than humans. Automated systems can be programmed to follow specific protocols and standards, ensuring consistent quality across all products. Robots are particularly beneficial in hazardous or physically demanding environments, enhancing safety and efficiency.
- Virtual Reality (VR) and Augmented Reality (AR): VR and AR technologies are being used to create immersive training simulations for quality control personnel. These simulations allow workers to experience real-world scenarios and learn best practices in a safe and controlled environment. VR and AR can also be used to enhance visual inspections, providing operators with detailed information and insights about product quality.
- Blockchain: Blockchain technology can be used to create secure and transparent records of product quality data, ensuring traceability and accountability throughout the supply chain. Blockchain can also be used to verify the authenticity of products and prevent counterfeiting.
Potential Future Technologies and Their Benefits
Technology | Benefits |
---|---|
Digital Twins | Creating virtual representations of physical assets and processes, enabling simulations and predictive maintenance to optimize quality and prevent defects. |
Biometric Authentication | Enhancing security and traceability by using unique biological characteristics to identify individuals and track product movement. |
Quantum Computing | Solving complex optimization problems and accelerating data analysis for advanced quality control algorithms and predictive modeling. |
Final Wrap-Up
As technology continues to evolve, the future of quality control holds even greater promise. Emerging technologies like blockchain, the Internet of Things (IoT), and augmented reality (AR) will further enhance quality control processes, enabling organizations to achieve new levels of efficiency, accuracy, and insight. By embracing these advancements, businesses can stay ahead of the curve and ensure that quality remains a core competitive advantage.
Technology is revolutionizing quality control in various industries, from manufacturing to healthcare. A prime example is the rise of autonomous store technology , where AI-powered systems manage inventory, track customer interactions, and even handle transactions. This automation not only enhances efficiency but also provides valuable data for improving product quality and customer service, highlighting the transformative power of technology in quality control.