Invisible Technologies Hiring AI Data Trainers
Invisible technologies hiring AI data trainers takes center stage as artificial intelligence seamlessly integrates into our lives. This shift towards “invisible” technologies, like AI, impacts how we perceive and adopt […]
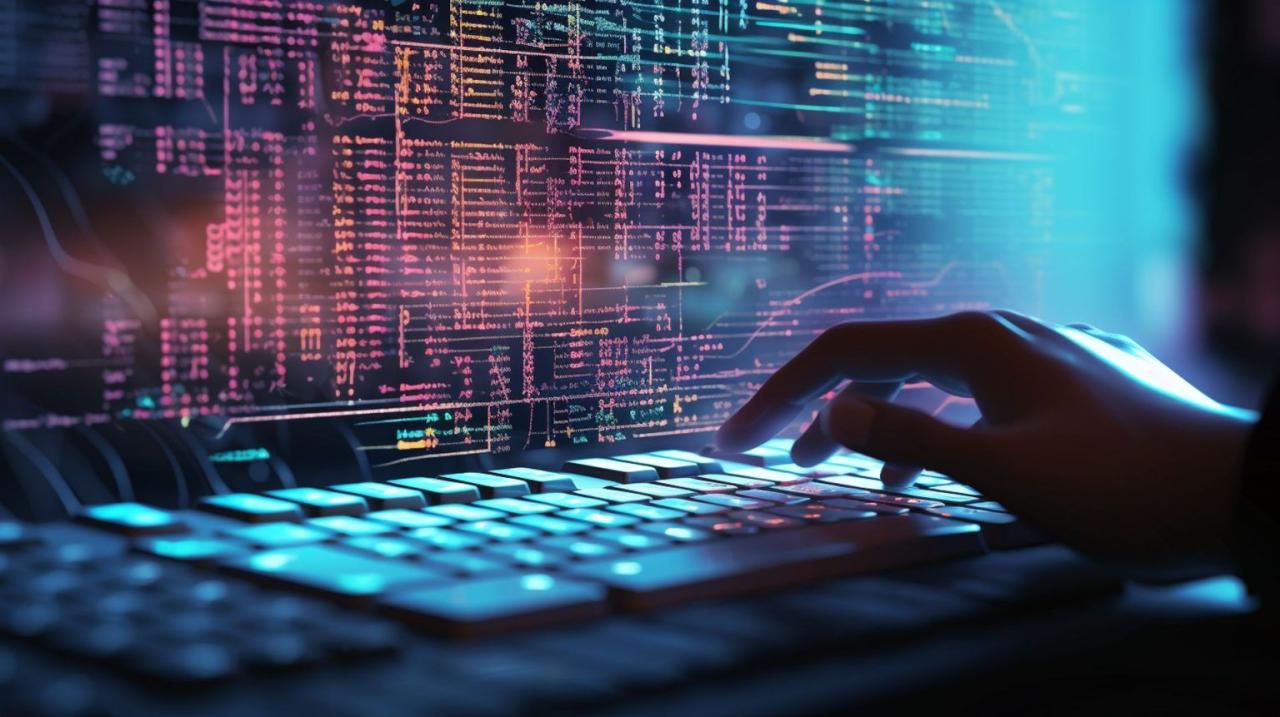
Invisible technologies hiring AI data trainers takes center stage as artificial intelligence seamlessly integrates into our lives. This shift towards “invisible” technologies, like AI, impacts how we perceive and adopt these advancements. From predictive algorithms in social media to personalized recommendations in e-commerce, the world is increasingly driven by unseen forces. AI data trainers play a crucial role in making these invisible technologies a reality, shaping the future of work and impacting our daily lives in ways we may not even realize.
The rise of invisible technologies presents both opportunities and challenges. Understanding the role of AI data trainers and the skills they possess is essential for navigating this evolving landscape. This article explores the complexities of AI data training, highlighting the importance of human oversight and ethical considerations as we move forward into a world increasingly powered by unseen forces.
Skills and Expertise Required for AI Data Training
AI data training is a crucial aspect of developing and deploying effective AI models. It involves preparing, cleaning, and labeling data to train AI algorithms, enabling them to learn and make accurate predictions. The demand for skilled AI data trainers is rapidly growing as businesses across industries are increasingly adopting AI solutions.
Domain Expertise
Domain expertise is essential for AI data trainers, particularly when working on projects within specific industries. A deep understanding of the industry’s context, terminology, and data sources allows trainers to make informed decisions about data selection, annotation, and quality control. For example, an AI data trainer working on a healthcare project would need to understand medical terminology, data privacy regulations, and the nuances of different healthcare datasets.
Technical Skills
AI data training requires a strong foundation in technical skills related to data analysis, machine learning, and programming.
- Data Analysis: AI data trainers must be proficient in data analysis techniques, including data cleaning, transformation, and visualization. They should be able to identify and handle missing data, outliers, and inconsistencies, ensuring data quality and integrity.
- Machine Learning: A solid understanding of machine learning concepts and algorithms is crucial. This includes familiarity with supervised learning, unsupervised learning, and reinforcement learning, as well as the ability to choose appropriate algorithms for specific tasks.
- Programming: Proficiency in programming languages commonly used in AI, such as Python, R, or Java, is essential. AI data trainers use these languages to develop scripts for data preprocessing, model training, and evaluation.
The Impact of AI Data Training on the Future of Work
The rapid advancement of artificial intelligence (AI) and its reliance on massive datasets for training have profound implications for the future of work. AI data training, the process of preparing and labeling data for AI models, is at the heart of this revolution, shaping the job market and raising ethical considerations.
Job Creation and Displacement
AI data training is creating new job opportunities in fields related to data annotation, data management, and AI model development. The demand for skilled professionals who can curate, clean, and label data is increasing as AI systems become more sophisticated. However, AI also has the potential to automate certain tasks currently performed by humans, leading to job displacement in specific sectors.
- For example, AI-powered chatbots are already replacing customer service representatives in some industries. Similarly, AI algorithms can automate tasks like data entry and document processing, potentially impacting roles in administrative and back-office functions.
Ethical Considerations in AI Data Training
The ethical implications of AI data training are crucial, as biased or incomplete datasets can lead to discriminatory outcomes.
- For instance, facial recognition systems trained on datasets predominantly featuring individuals of a specific race or gender can exhibit bias, leading to inaccurate or unfair results.
- Ensuring data diversity and representation in AI training datasets is essential to mitigate bias and promote fairness in AI applications.
Human-in-the-Loop Approaches in AI
Human-in-the-loop (HITL) approaches are gaining prominence in AI systems, recognizing the importance of human oversight and intervention.
- In HITL systems, humans play a crucial role in validating AI outputs, providing feedback, and ensuring ethical considerations are met.
- For example, in medical diagnosis, AI systems can assist doctors by analyzing medical images and providing insights. However, final diagnosis and treatment decisions still require human expertise and judgment.
“The future of work will be shaped by a combination of human and AI capabilities, where humans focus on higher-level tasks requiring creativity, critical thinking, and ethical decision-making, while AI automates routine tasks and enhances productivity.”
The Future of AI Data Training
The field of AI data training is rapidly evolving, driven by advancements in technology, changing data landscapes, and a growing awareness of the ethical implications of AI. As AI becomes more sophisticated and integrated into various aspects of our lives, the demand for high-quality training data will continue to rise, shaping the future of this critical field.
Advancements in Data Annotation Techniques
The accuracy and effectiveness of AI models are directly influenced by the quality of training data. Advancements in data annotation techniques are crucial for improving the efficiency and accuracy of AI training. Here are some key trends:
- Automated Annotation: Machine learning algorithms are increasingly used to automate the annotation process, reducing the reliance on manual labeling. This can significantly accelerate the annotation process, especially for large datasets. For instance, tools that leverage natural language processing (NLP) can automatically label text data based on predefined categories, while image annotation tools can use object detection algorithms to identify and label objects in images.
- Active Learning: Active learning techniques aim to optimize the annotation process by intelligently selecting the most informative data points for human annotators. This helps to prioritize the most critical data, reducing the overall annotation effort. For example, an active learning algorithm might identify ambiguous data points that require further human review, ensuring that the training data is as accurate as possible.
- Federated Learning: Federated learning allows training models on decentralized data sources without requiring the data to be centralized. This addresses privacy concerns by enabling data to be trained locally, minimizing the need to share sensitive information. For example, a healthcare AI model could be trained on data from multiple hospitals without requiring them to share their patients’ data.
The Growing Importance of Data Privacy and Security, Invisible technologies hiring ai data trainer
As AI systems become more powerful and pervasive, data privacy and security become paramount. The use of personal data for training AI models raises ethical concerns, and regulatory frameworks are being developed to address these concerns.
- Data Minimization: Using only the necessary data for training AI models is crucial to minimizing the risk of privacy breaches. This involves carefully considering the purpose of the model and ensuring that only the relevant data is collected and used.
- Differential Privacy: Differential privacy techniques add noise to data to make it difficult to identify individuals, while still preserving the overall statistical properties of the data. This helps to protect the privacy of individuals whose data is used for training AI models. For example, a company using customer data to train a recommendation engine could use differential privacy to protect the identity of individual customers.
- Data Encryption: Encrypting data during storage and transmission is essential to prevent unauthorized access and data breaches. This is especially important when handling sensitive data, such as personal health information or financial data.
The Role of Ethical Considerations
The development and deployment of AI systems must be guided by ethical principles to ensure that they are used responsibly and benefit society. Ethical considerations are crucial in shaping the future of AI data training, particularly in relation to:
- Bias Mitigation: AI models can inherit biases from the training data they are exposed to. It is crucial to identify and mitigate biases in training data to ensure that AI systems are fair and equitable. For instance, a facial recognition system trained on a dataset with a disproportionate number of individuals from a specific demographic group could exhibit bias in its performance.
- Transparency and Explainability: AI models can be complex and difficult to understand. Ensuring transparency and explainability in AI systems is crucial for building trust and accountability. This involves developing methods to understand how AI models make decisions and providing explanations for their outputs. For example, a loan approval system should be able to explain why a loan application was approved or denied, rather than simply providing a binary decision.
- Accountability: Establishing clear lines of accountability for the actions of AI systems is essential. This involves identifying who is responsible for the decisions made by AI systems and ensuring that there are mechanisms for addressing any potential harm caused by AI. For example, if an autonomous vehicle causes an accident, it is important to determine who is liable for the accident and how to address the consequences.
Final Wrap-Up: Invisible Technologies Hiring Ai Data Trainer
As we delve deeper into the world of invisible technologies, the demand for skilled AI data trainers will only increase. The future of work will be shaped by their ability to train and refine AI systems, ensuring their accuracy, fairness, and ethical use. Understanding the role of AI data trainers and the impact of their work is crucial for navigating this exciting and complex future.
The world of invisible technologies is constantly evolving, and with it, the need for AI data trainers who can understand and interpret complex datasets. These trainers play a crucial role in shaping the future of everything from self-driving cars to medical advancements, like extracorporeal pulse activation technology , which uses targeted pulses to stimulate healing.
As the field of invisible technologies expands, the demand for skilled AI data trainers will only continue to grow, ensuring that these groundbreaking innovations reach their full potential.