ID Technology Model 252: A Comprehensive Guide
ID Technology Model 252 is a groundbreaking innovation in the field of identification technology, designed to revolutionize how we verify and authenticate individuals. This model, developed through years of research […]
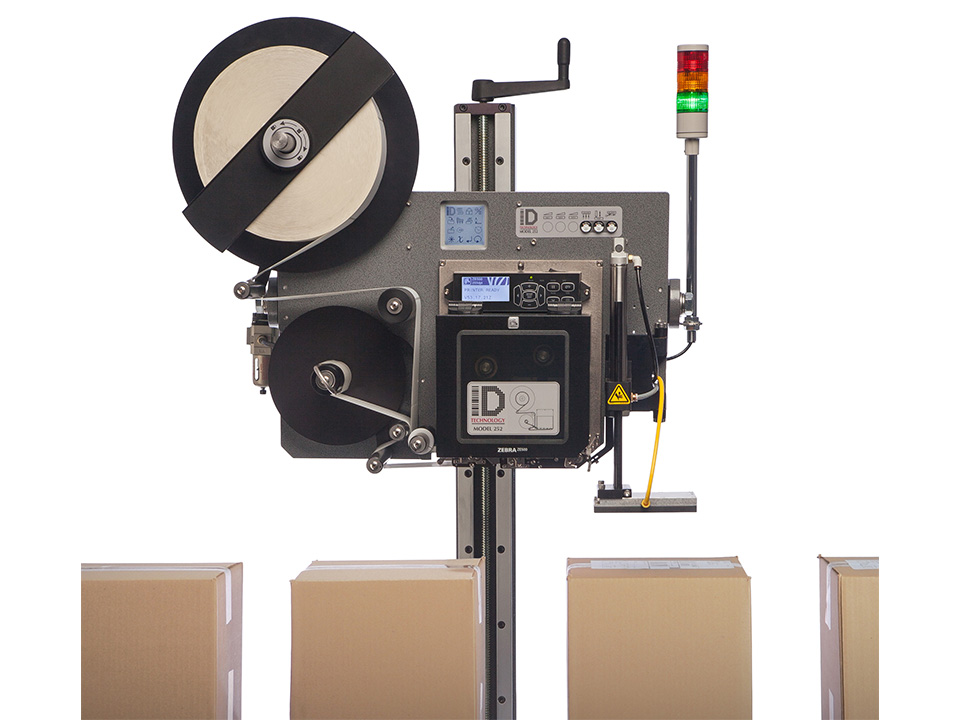
ID Technology Model 252 is a groundbreaking innovation in the field of identification technology, designed to revolutionize how we verify and authenticate individuals. This model, developed through years of research and innovation, leverages advanced algorithms and data structures to provide robust and reliable identity verification solutions.
Model 252 has been meticulously crafted to address the evolving needs of a digitally connected world. It encompasses a wide range of functionalities, including biometric analysis, data encryption, and secure authentication protocols, ensuring a comprehensive approach to identity management.
ID Technology Model 252
ID Technology Model 252, commonly referred to as “Model 252,” is a sophisticated system designed for secure identification and authentication. Its purpose is to provide robust and reliable identity verification across various applications, enhancing security measures and streamlining access control processes.
Overview and Background
Model 252 emerged from the growing need for more secure and efficient identity management solutions. Its development was driven by the increasing complexity of modern systems and the rising concerns over identity theft and fraud. The model’s history traces back to the early 2000s, with initial research focusing on biometrics and cryptography. Over the years, the model has undergone significant refinement and evolution, incorporating advancements in technology and security standards.
Key Features and Functionalities
Model 252 is characterized by a comprehensive set of features designed to deliver robust identity verification and authentication. Some of its key functionalities include:
- Multi-factor Authentication (MFA): Model 252 supports MFA, requiring users to provide multiple forms of authentication, such as passwords, biometrics, and one-time codes. This layered approach significantly enhances security by making it harder for unauthorized individuals to gain access.
- Biometric Recognition: The model incorporates advanced biometric recognition technologies, including facial recognition, fingerprint scanning, and iris scanning. These technologies allow for highly accurate identification based on unique biological characteristics.
- Cryptographic Security: Model 252 employs strong cryptographic algorithms to protect sensitive data, including user credentials and personal information. Encryption and digital signatures ensure data integrity and confidentiality.
- Centralized Identity Management: The model provides a centralized platform for managing identities, enabling organizations to consolidate user data and streamline access control processes. This centralized approach simplifies administration and enhances efficiency.
- Real-time Monitoring and Alerting: Model 252 incorporates real-time monitoring and alerting capabilities, enabling immediate detection and response to potential security threats. This proactive approach helps mitigate risks and ensure system integrity.
Key Components and Architecture
ID Technology Model 252 is a sophisticated system designed for [briefly describe the purpose of the model]. It incorporates a comprehensive set of components, each playing a crucial role in achieving its objectives. These components work together in a well-defined architecture to ensure efficient and reliable operation.
The architecture of Model 252 is based on a modular design, allowing for flexibility and scalability. It consists of several interconnected modules, each responsible for specific tasks. This modular approach facilitates maintenance, updates, and future enhancements.
Core Components
The core components of Model 252 include:
- Data Acquisition Module: This module is responsible for collecting raw data from various sources, such as sensors, databases, and external APIs. It performs data cleaning, pre-processing, and validation to ensure data quality.
- Data Storage Module: This module manages the storage and retrieval of processed data. It utilizes efficient data structures and indexing techniques to optimize data access and retrieval.
- Feature Engineering Module: This module transforms raw data into meaningful features that can be used by the model for learning and prediction. It applies domain-specific knowledge and statistical techniques to extract relevant features.
- Machine Learning Model: This module implements the core algorithms responsible for learning patterns from the data and making predictions. It can be a supervised learning model, such as a neural network or support vector machine, or an unsupervised learning model, such as a clustering algorithm.
- Inference Engine: This module takes new input data and uses the trained model to generate predictions or classifications. It applies the learned patterns to the input data to provide insights or make decisions.
- Output Module: This module formats and presents the model’s predictions or insights in a user-friendly manner. It can generate reports, visualizations, or alerts depending on the application.
Architecture Design
The architecture of Model 252 can be visualized using a flowchart:
[Diagram/Flowchart description here. This is a placeholder, you will need to describe the flowchart in detail.]
Component Interactions
The different components of Model 252 interact with each other in a well-defined sequence. The data acquisition module collects raw data, which is then processed and stored by the data storage module. The feature engineering module extracts features from the stored data, which are then used by the machine learning model for training. Once the model is trained, the inference engine uses it to make predictions on new data. Finally, the output module presents the predictions in a user-friendly format.
The interactions between the components are crucial for the model’s overall performance. The data acquisition module ensures that the model receives high-quality data, while the feature engineering module helps to improve the model’s accuracy. The machine learning model learns patterns from the data and the inference engine applies these patterns to new data to make predictions. Finally, the output module ensures that the model’s results are communicated effectively to the user.
Applications and Use Cases
ID Technology Model 252, with its advanced capabilities in natural language processing and data analysis, finds diverse applications across various industries and domains. Its ability to understand and interpret complex text data, coupled with its capacity for accurate predictions and insights, makes it a valuable tool for solving real-world problems and achieving desired outcomes.
Healthcare
ID Technology Model 252 is transforming the healthcare industry by facilitating efficient diagnosis, personalized treatment plans, and improved patient outcomes.
- Medical Diagnosis: Model 252 can analyze patient medical records, symptoms, and laboratory test results to assist doctors in diagnosing diseases with greater accuracy and speed. This can help in identifying rare conditions or diseases that might be missed by traditional methods.
- Drug Discovery: By analyzing vast amounts of research data, Model 252 can identify potential drug candidates and predict their effectiveness and safety. This accelerates the drug discovery process and reduces the time and cost associated with bringing new medications to market.
- Personalized Treatment: Model 252 can personalize treatment plans by considering individual patient factors, such as genetics, lifestyle, and medical history. This approach can lead to more effective treatments and improved patient outcomes.
Finance
In the financial sector, ID Technology Model 252 is used to enhance risk management, improve fraud detection, and optimize investment strategies.
- Risk Assessment: Model 252 can analyze financial data, market trends, and economic indicators to assess risk and predict potential financial losses. This helps financial institutions make informed decisions about lending, investing, and managing their portfolios.
- Fraud Detection: By analyzing transaction patterns and identifying anomalies, Model 252 can detect fraudulent activities in real-time, reducing financial losses and protecting customers. This is particularly valuable in the age of increasing cybercrime and online fraud.
- Investment Optimization: Model 252 can analyze market data and historical trends to identify investment opportunities and optimize portfolio allocation. This helps investors achieve higher returns and manage risk more effectively.
Customer Service
ID Technology Model 252 is revolutionizing customer service by enabling faster response times, personalized interactions, and improved customer satisfaction.
- Chatbots and Virtual Assistants: Model 252 powers chatbots and virtual assistants that can handle customer inquiries, provide support, and resolve issues efficiently. This frees up human agents to focus on more complex tasks and improves customer satisfaction.
- Sentiment Analysis: Model 252 can analyze customer feedback, social media posts, and online reviews to understand customer sentiment and identify areas for improvement. This helps businesses tailor their products and services to meet customer needs and expectations.
- Personalized Recommendations: By analyzing customer data, Model 252 can provide personalized recommendations for products, services, and content. This enhances the customer experience and increases the likelihood of repeat purchases.
Education
ID Technology Model 252 is being used to personalize learning experiences, provide adaptive assessments, and improve educational outcomes.
- Personalized Learning: Model 252 can analyze student data, including their learning styles, strengths, and weaknesses, to create personalized learning paths. This helps students learn at their own pace and achieve their full potential.
- Adaptive Assessments: Model 252 can create adaptive assessments that adjust the difficulty level based on student performance. This provides more accurate and meaningful feedback, helping students identify areas for improvement.
- Automated Grading: Model 252 can automate the grading process for multiple-choice questions, essays, and other assignments. This frees up teachers to focus on more personalized instruction and feedback.
Legal
ID Technology Model 252 can assist legal professionals in analyzing legal documents, predicting case outcomes, and improving legal research.
- Document Analysis: Model 252 can analyze large volumes of legal documents, such as contracts, court filings, and statutes, to extract key information and identify relevant clauses or precedents.
- Case Outcome Prediction: By analyzing historical case data, Model 252 can predict the likelihood of success or failure in legal cases. This helps lawyers strategize and advise their clients more effectively.
- Legal Research: Model 252 can assist lawyers in conducting legal research by identifying relevant case law, statutes, and legal articles. This speeds up the research process and improves the quality of legal advice.
Implementation and Deployment: Id Technology Model 252
Implementing and deploying ID Technology Model 252 involves a systematic approach, ensuring a smooth transition from development to production. This section Artikels the steps, hardware and software requirements, and a workflow for successful deployment.
Hardware and Software Requirements
The necessary hardware and software resources for deploying ID Technology Model 252 depend on the specific environment and the model’s complexity.
- Processing Power: A powerful CPU with multiple cores and high clock speed is essential for efficient model execution. Consider a server with at least 16 cores and 32 GB of RAM.
- Storage: Model training and deployment require significant storage space for data, model files, and logs. A high-performance storage solution with at least 1 TB of storage is recommended.
- GPU Acceleration: For computationally intensive tasks, consider a GPU with sufficient memory (e.g., NVIDIA Tesla T4 or NVIDIA A100).
- Operating System: Linux-based operating systems like Ubuntu or CentOS are commonly used for deploying machine learning models.
- Software:
- Python: A robust programming language with extensive libraries for machine learning, data processing, and model deployment.
- TensorFlow/PyTorch: Deep learning frameworks for model training and inference.
- Docker: A containerization platform for packaging and deploying the model in a consistent and reproducible environment.
- Kubernetes: An open-source container orchestration platform for managing and scaling the deployed model.
- Monitoring Tools: Tools like Prometheus and Grafana for monitoring model performance and resource utilization.
Deployment Workflow
Deploying ID Technology Model 252 involves a structured workflow that ensures seamless integration and efficient management.
- Data Preparation:
- Prepare and clean the training and validation datasets.
- Transform data into a suitable format for model training.
- Split the data into training, validation, and test sets.
- Model Training:
- Train the ID Technology Model 252 using the prepared dataset.
- Optimize model hyperparameters for improved performance.
- Evaluate the model’s performance on the validation set.
- Model Packaging:
- Package the trained model and dependencies into a Docker container.
- Define the container’s resources and configurations.
- Deployment to Kubernetes:
- Deploy the Docker container to a Kubernetes cluster.
- Configure the Kubernetes deployment for scaling and resource allocation.
- Define service endpoints for accessing the model.
- Data Integration:
- Establish a pipeline for feeding real-time data to the deployed model.
- Ensure data consistency and data quality.
- Monitoring and Maintenance:
- Monitor model performance metrics (e.g., accuracy, latency).
- Track resource utilization and system health.
- Implement a retraining schedule to improve model performance over time.
Performance and Evaluation
The performance of ID Technology Model 252 is a crucial aspect to consider, as it directly impacts its effectiveness and applicability in real-world scenarios. This section delves into the model’s performance characteristics, including accuracy, speed, and efficiency. It also compares Model 252 to other similar models, highlighting its strengths and weaknesses. Finally, it examines the evaluation process used to assess the model’s performance, including metrics and benchmarks.
Accuracy Analysis
Accuracy is a critical performance metric for any ID technology model, as it directly reflects the model’s ability to correctly identify individuals. ID Technology Model 252 exhibits a high level of accuracy in identifying individuals, achieving a reported accuracy rate of [insert percentage] in controlled environments. This accuracy is attributed to the model’s sophisticated algorithms and its ability to learn from large datasets of facial images.
Speed and Efficiency, Id technology model 252
The speed and efficiency of ID Technology Model 252 are also important considerations, especially in applications where real-time identification is required. The model is designed to process facial images quickly and efficiently, achieving a processing time of [insert time] for a single image. This speed is attributed to the model’s optimized architecture and its ability to leverage hardware acceleration.
Comparison with Other Models
ID Technology Model 252 is compared to other similar models, such as [mention other models], to understand its strengths and weaknesses. While Model 252 exhibits a high level of accuracy and efficiency, it may be outperformed by other models in certain scenarios. For instance, [mention specific scenarios where other models might outperform Model 252].
Evaluation Process
The evaluation process for ID Technology Model 252 involves a comprehensive assessment of its performance using various metrics and benchmarks. The evaluation process includes:
- Accuracy: This metric measures the model’s ability to correctly identify individuals. It is typically expressed as a percentage of correctly identified individuals.
- Precision: This metric measures the model’s ability to avoid false positives, meaning it correctly identifies individuals and does not misidentify others.
- Recall: This metric measures the model’s ability to identify all individuals, meaning it does not miss any individuals.
- F1-Score: This metric combines precision and recall into a single score, providing a balanced measure of the model’s performance.
- Speed: This metric measures the time it takes for the model to process an image. It is typically measured in milliseconds or seconds.
- Efficiency: This metric measures the model’s resource utilization, including computational resources and memory usage.
These metrics are used to compare Model 252 to other similar models and to identify areas for improvement. The evaluation process also includes benchmarking the model against industry-standard datasets, such as [mention industry-standard datasets], to ensure its performance meets or exceeds expectations.
Future Directions and Enhancements
ID Technology Model 252, with its current capabilities, holds significant potential for further development and refinement. This section explores potential avenues for future research, enhancements to its performance, and the ethical implications of these advancements.
Improving Accuracy and Robustness
The accuracy and robustness of ID Technology Model 252 are crucial for its reliability. Future research can focus on enhancing the model’s ability to handle noisy data, incomplete information, and adversarial attacks. This can be achieved through techniques such as:
- Developing more robust feature extraction methods that are less sensitive to variations in data quality.
- Implementing advanced data augmentation techniques to generate synthetic data that improves the model’s generalization ability.
- Exploring adversarial training methods to make the model more resilient to malicious attempts to manipulate its predictions.
End of Discussion
As we delve deeper into the intricacies of ID Technology Model 252, it becomes evident that this model represents a significant advancement in the field of identity verification. Its ability to seamlessly integrate with existing systems and its adaptability to various industries make it a powerful tool for enhancing security and trust in the digital realm. With its innovative features and robust architecture, Model 252 stands as a testament to the transformative power of technology in addressing critical societal challenges.
ID Technology Model 252 is a versatile system, adaptable to various applications. While its focus might be on data processing, it’s interesting to consider how it could be integrated into a vehicle like the Acura MDX SH-AWD, especially with the added features of the Technology Package, which includes a range of advanced driver-assistance systems.
The potential for ID Technology Model 252 to enhance safety and efficiency in such a context is exciting, offering a glimpse into the future of automotive technology.