Demand Technology: Shaping the Future of Business
Demand technology has revolutionized how businesses operate, from forecasting sales to optimizing resource allocation. It encompasses a range of tools and techniques that leverage data analysis, AI, and machine learning […]
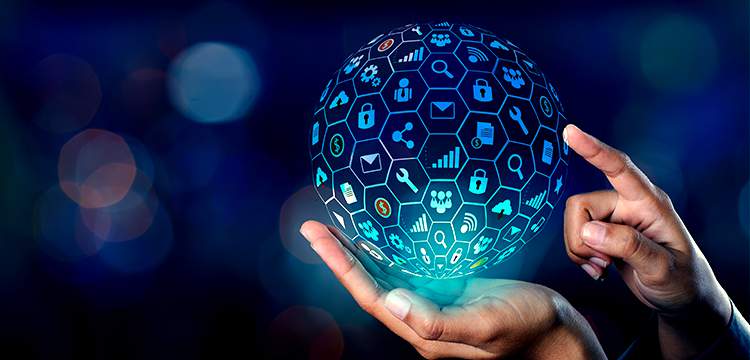
Demand technology has revolutionized how businesses operate, from forecasting sales to optimizing resource allocation. It encompasses a range of tools and techniques that leverage data analysis, AI, and machine learning to understand and manage demand effectively.
The evolution of demand technology has been driven by the need to navigate increasingly complex market dynamics. Early methods relied on simple forecasting models, but as businesses grew and markets became more volatile, sophisticated solutions emerged. These advancements have enabled businesses to make more accurate predictions, improve inventory management, and enhance customer experiences.
The Evolution of Demand Technology
Demand technology has evolved significantly over time, reflecting the changing needs of businesses and the increasing complexity of the market. From rudimentary forecasting methods to sophisticated AI-powered solutions, the journey of demand technology has been marked by innovation and a relentless pursuit of accuracy and efficiency.
Early Stages: Forecasting Methods
The earliest demand technology solutions were primarily based on simple forecasting methods. These methods relied on historical data and basic statistical analysis to predict future demand. While these methods were useful for initial demand planning, they often lacked the sophistication to accurately capture the nuances of complex market dynamics.
- Moving Average: This method calculates the average demand over a specific period to predict future demand. It is simple to implement but may not be effective for products with seasonal or cyclical patterns.
- Exponential Smoothing: This method assigns more weight to recent data points, making it more responsive to changes in demand. It is more accurate than moving average but still relies heavily on historical data.
The Rise of Statistical Modeling
With the advent of computers and the development of statistical modeling techniques, demand technology took a significant leap forward. Statistical models allowed for more sophisticated analysis of historical data, enabling businesses to develop more accurate forecasts and make better demand planning decisions.
- Regression Analysis: This technique identifies the relationship between demand and various factors like price, promotions, and seasonality. It allows businesses to predict demand based on these factors.
- Time Series Analysis: This method analyzes historical demand data to identify patterns and trends, which can be used to forecast future demand. It is particularly useful for products with seasonal or cyclical demand patterns.
The Integration of Technology
As technology advanced, demand technology solutions became more integrated with other business systems, such as enterprise resource planning (ERP) and customer relationship management (CRM). This integration enabled businesses to access real-time data from various sources, improving the accuracy and responsiveness of demand forecasts.
- ERP Integration: This integration allows businesses to access real-time data on inventory levels, production schedules, and customer orders, which can be used to improve demand forecasts.
- CRM Integration: This integration provides access to customer data, such as purchase history, preferences, and demographics, which can be used to personalize demand forecasts and marketing campaigns.
The Age of AI and Machine Learning
The most recent evolution of demand technology has been driven by the rise of artificial intelligence (AI) and machine learning (ML). These technologies enable businesses to analyze vast amounts of data from various sources, including social media, web traffic, and sensor data, to generate more accurate and insightful demand forecasts.
- Predictive Analytics: AI and ML algorithms can analyze historical data and identify patterns and trends that are not easily detected by traditional statistical models. This allows for more accurate and timely demand forecasts.
- Demand Sensing: AI and ML can monitor real-time data from various sources, such as social media sentiment, search engine trends, and weather data, to identify emerging demand patterns and adjust forecasts accordingly.
Key Components of Demand Technology
Demand technology encompasses a suite of tools and techniques that enable businesses to understand, predict, and manage customer demand effectively. These components work in tandem to provide a comprehensive view of demand patterns, allowing organizations to make informed decisions and optimize their operations.
Data Collection
Data collection forms the foundation of demand technology, providing the raw material for analysis and forecasting. Businesses gather data from various sources, including:
- Sales Transactions: Historical sales data provides insights into past demand patterns, including product performance, seasonality, and customer buying behavior.
- Customer Relationship Management (CRM) Systems: CRM systems store customer information, including purchase history, preferences, and demographics, which can be used to segment customers and tailor marketing efforts.
- Web Analytics: Website traffic data reveals user behavior, product views, and conversion rates, providing valuable insights into customer engagement and online demand.
- Social Media Monitoring: Social media platforms offer valuable data on brand sentiment, customer feedback, and trending topics, enabling businesses to understand public perception and anticipate demand shifts.
- Market Research: Conducting surveys, focus groups, and market research studies provides insights into consumer preferences, market trends, and competitive landscape.
Data Analysis
Once collected, data needs to be analyzed to extract meaningful insights. Data analysis techniques used in demand technology include:
- Descriptive Analytics: Descriptive analytics summarizes historical data, identifying trends, patterns, and outliers. This helps businesses understand past demand behavior and identify key drivers.
- Predictive Analytics: Predictive analytics uses statistical models and machine learning algorithms to forecast future demand based on historical data and external factors. This enables businesses to anticipate demand fluctuations and make proactive adjustments.
- Prescriptive Analytics: Prescriptive analytics goes beyond forecasting by recommending actions to optimize demand management. It leverages data and algorithms to suggest optimal pricing strategies, inventory levels, and marketing campaigns.
Demand Forecasting
Demand forecasting is a crucial component of demand technology, enabling businesses to predict future demand with reasonable accuracy. Forecasting methods used include:
- Time Series Analysis: Time series analysis uses historical demand data to identify patterns and trends, extrapolating these patterns into the future to create forecasts.
- Causal Forecasting: Causal forecasting considers external factors that influence demand, such as economic conditions, competitor actions, and promotional campaigns. It uses statistical models to incorporate these factors into forecasts.
- Machine Learning Forecasting: Machine learning algorithms can learn from complex patterns in historical data and external factors to generate highly accurate demand forecasts. These models can adapt to changing market conditions and provide more reliable predictions.
Demand Optimization
Demand optimization focuses on aligning supply and demand to maximize profitability and customer satisfaction. This involves:
- Inventory Management: Demand forecasting helps businesses optimize inventory levels, minimizing stockouts and excess inventory. This reduces storage costs and ensures timely product availability.
- Pricing Strategies: Demand insights can inform dynamic pricing strategies, adjusting prices based on demand fluctuations, competition, and customer willingness to pay. This maximizes revenue and profitability.
- Marketing and Sales: Demand forecasts enable businesses to target marketing efforts effectively, allocating resources to products and customer segments with the highest potential demand. This increases conversion rates and customer acquisition.
- Production Planning: Demand forecasts guide production planning, ensuring that manufacturing capacity is aligned with anticipated demand. This minimizes production delays and ensures timely product delivery.
Key Components of Demand Technology
Component | Functionality |
---|---|
Data Collection | Gathering data from various sources to provide insights into past and current demand patterns. |
Data Analysis | Extracting meaningful insights from collected data through descriptive, predictive, and prescriptive analytics. |
Demand Forecasting | Predicting future demand based on historical data and external factors using time series analysis, causal forecasting, and machine learning. |
Demand Optimization | Aligning supply and demand to maximize profitability and customer satisfaction through inventory management, pricing strategies, marketing and sales optimization, and production planning. |
Benefits and Challenges of Implementing Demand Technology
Implementing demand technology can bring about significant improvements in various aspects of a business, leading to enhanced efficiency, cost savings, and customer satisfaction. However, the implementation process itself comes with its own set of challenges that need to be carefully considered and addressed.
Benefits of Implementing Demand Technology
Demand technology offers a wide range of benefits that can significantly impact a business’s performance and profitability. By leveraging data and analytics, businesses can gain valuable insights into customer behavior, optimize their operations, and make informed decisions.
- Improved Forecasting Accuracy: Demand technology leverages historical data and advanced algorithms to generate accurate forecasts of future demand. This allows businesses to anticipate fluctuations in demand, optimize inventory levels, and reduce the risk of stockouts or overstocking. For example, a retail company can use demand technology to predict seasonal demand for specific products, ensuring that they have adequate inventory available during peak periods.
- Reduced Inventory Costs: By accurately forecasting demand, businesses can minimize the amount of inventory they need to hold, leading to significant cost savings. This is particularly important for businesses with high inventory carrying costs, such as those in the manufacturing or retail sectors. For instance, a manufacturing company can use demand technology to optimize its production schedule, reducing the need to hold large amounts of raw materials and finished goods in inventory.
- Optimized Resource Allocation: Demand technology provides insights into resource utilization, allowing businesses to allocate resources more efficiently. By understanding demand patterns, businesses can ensure that they have the right resources available at the right time. For example, a call center can use demand technology to predict call volume and staff accordingly, ensuring that they have enough agents available to handle incoming calls.
- Enhanced Customer Satisfaction: Demand technology enables businesses to better understand customer needs and preferences. By analyzing customer data, businesses can personalize their offerings and provide a more tailored customer experience. For example, an e-commerce company can use demand technology to recommend products based on a customer’s past purchases and browsing history.
Challenges of Implementing Demand Technology
While demand technology offers numerous benefits, implementing it can present significant challenges. Businesses need to carefully consider these challenges and develop strategies to overcome them.
- Data Quality: The accuracy of demand forecasts relies heavily on the quality of the data used to train the algorithms. Businesses need to ensure that their data is accurate, complete, and consistent. This may involve data cleansing, validation, and integration processes. For example, a company may need to invest in data quality tools to identify and correct errors in its customer data, ensuring that the data used for demand forecasting is reliable.
- System Integration: Integrating demand technology with existing systems can be complex and time-consuming. Businesses need to ensure that the technology seamlessly integrates with their existing IT infrastructure, including enterprise resource planning (ERP) systems, customer relationship management (CRM) systems, and supply chain management (SCM) systems. For instance, a company may need to invest in middleware solutions to facilitate data exchange between different systems.
- Workforce Training: Implementing demand technology requires training employees to use the new systems and interpret the data generated. Businesses need to invest in training programs to ensure that employees have the skills and knowledge to effectively utilize the technology. For example, a company may need to provide training to its sales team on how to use demand forecasting data to optimize their sales strategies.
Comparison of Benefits and Challenges
Benefits | Challenges |
---|---|
Improved Forecasting Accuracy | Data Quality |
Reduced Inventory Costs | System Integration |
Optimized Resource Allocation | Workforce Training |
Enhanced Customer Satisfaction |
Ultimate Conclusion: Demand Technology
In conclusion, demand technology is a powerful tool that empowers businesses to make data-driven decisions and gain a competitive edge. By embracing its potential, companies can optimize operations, enhance customer satisfaction, and drive sustainable growth. As technology continues to evolve, we can expect even more innovative solutions that will shape the future of demand management.
Demand technology is constantly evolving, impacting various industries. In the healthcare sector, this evolution is evident in the rise of chiropractic technology , which offers innovative solutions for patient care and practice management. These advancements, in turn, further fuel the demand for sophisticated technology, creating a cyclical relationship between technology and its application in the healthcare field.